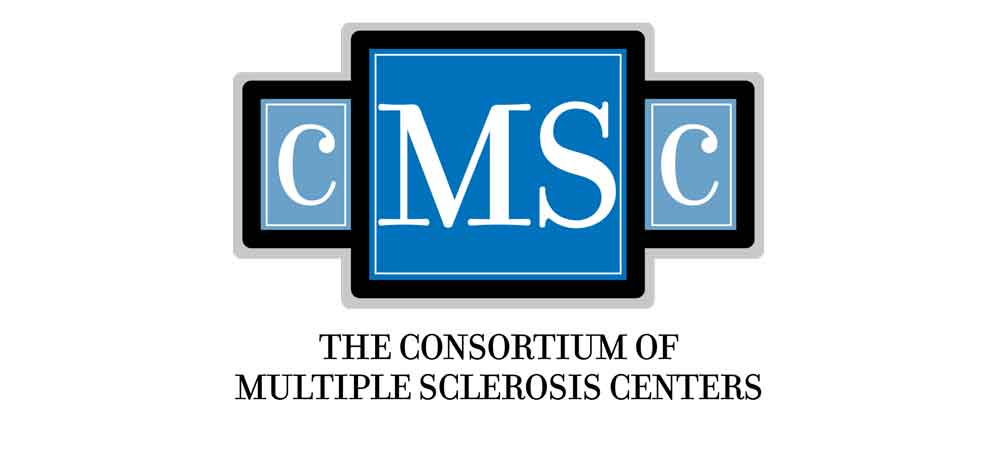
Machine learning framework for multiple sclerosis risk stratification and healthcare cost prediction
2024 CMSC Conference Presentation
Lukasz S. Wylezinski1,2, Jamieson D. Gray1, and Charles F. Spurlock, III1,2,3
1 Decode Health, Nashville, TN, USA 37203
2 Department of Medicine, Vanderbilt University School of Medicine, Nashville, TN 37203
3 Wagner School of Public Service, New York University, New York, NY 10012
Background: Multiple sclerosis (MS) is a chronic, progressive autoimmune disease resulting in irreversible neurological damage that leads to deteriorating patient quality of life and increasing economic burden on the patient, their families, and the healthcare system. Early diagnosis, treatment, and effective disease monitoring are imperative to reduce long-term patient disability.
Objectives: This study aimed to develop ML algorithms that utilize healthcare insurance claims datasets to accurately predict MS patients at high risk for the largest monthly healthcare spend.
Methods: Novel methods to leverage emerging, large-scale healthcare datasets provide a unique opportunity to understand healthcare patterns preceding acute emergencies, loss of drug efficacy, or general deterioration of health that can allow for timely interventions and stratification of patients based on emergent risk. Machine learning (ML) methods can efficiently interrogate large healthcare datasets to predict disease risk, healthcare spending, and risk stratify patients to help care teams personalize treatment plans and improve outcomes.
Results: ML algorithms most accurately predicted the high-risk, high-spending group of MS patients compared to other historical spending assessments. ML methods identified a significantly larger proportion of the high-risk top-spending individuals, particularly new entrants into this group, each month compared to other approaches. Applying this ML framework to an out-of-sample dataset predicted, on average, 76.0% of the total top ten percent of actual healthcare spend, while alternative methods using historical four-month and prior-month spending only identified 43.5% and 36.5%, respectively.
Conclusions: Our findings support the hypothesis that ML approaches applied to insurance claims data can accurately predict MS patients at risk of the highest monthly healthcare costs, helping to prioritize these individuals for care. This disease-agnostic ML framework can be applied to other autoimmune diseases to identify patients who may benefit most from immediate care and track specific healthcare utilization patterns to optimize healthcare delivery.