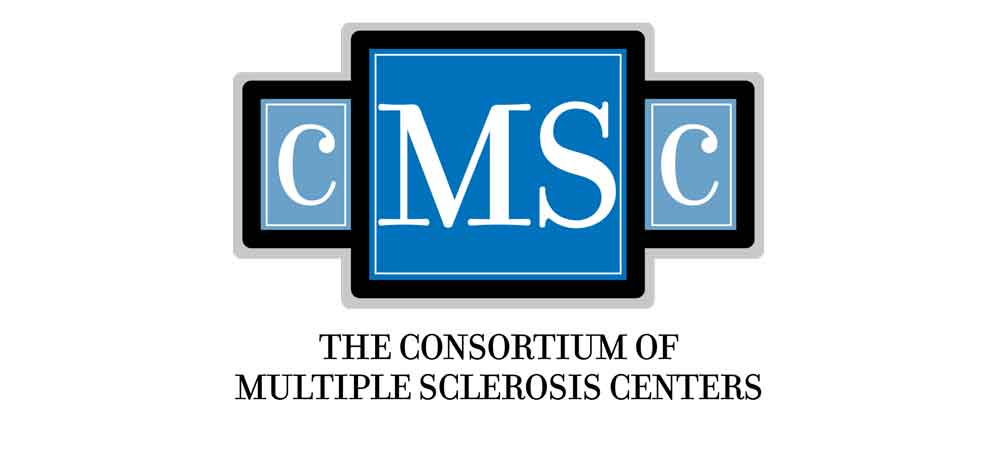
Advanced multivariate analysis to discover candidate RNA biomarkers to distinguish relapsing-remitting multiple sclerosis and neuromyelitis optica
2024 CMSC Conference Presentation
Lukasz S. Wylezinski1,2, Cheryl L. Sesler1, Jamieson D. Gray1, Guzel I. Shaginurova1, Elena V. Grigorenko1, Franklin R. Cockerill, III1,3,4, Michael K. Racke5, Charles F. Spurlock, III1,2,6
1 Decode Health, Nashville, TN, USA 37203
2 Department of Medicine, Vanderbilt University School of Medicine, Nashville, TN 37203
3 Department of Medicine, Rush University Medical Center, Chicago, IL 60612
4Trusted Health Advisors, Scottsdale, AZ 85251
5 Quest Diagnostics, Secaucus, NJ 07094
6 Wagner School of Public Health, New York University, New York, NY 10012
Multiple sclerosis (MS) is a chronic demyelinating disease causing irreversible neurological damage. Differential diagnosis from other inflammatory neurologic diseases, such as neuromyelitis optica (NMO), is challenging due to shared clinical signs and symptoms. Large, transcriptomic studies coupled with advanced data analytics can transform the understanding of complex disease and lead to improved biomarker discovery for clinical applications. We sought to identify candidate RNA biomarkers to distinguish relapsing-remitting MS (RRMS) and NMO using multivariate approaches. Total RNA sequencing was performed using whole blood collected into PAXgene Blood RNA tubes from patients with RRMS (n=105) or NMO (n=53) and healthy controls (HCs, n=69). RRMS and NMO patients were treatment-naïve and myelin oligodendrocyte glycoprotein antibody negative. Sequences were aligned to the human genome and quantified. Advanced analytics, including differential gene expression, competitive machine learning (ML), and consensus non-negative matrix factorization (cNMF), were leveraged in the following case/control comparisons: NMO/RRMS, RRMS/All Others (NMO+HC), and NMO/All Others (RRMS+HC). Genes distinguishing RRMS and NMO across all independent methods (candidate biomarkers) were interrogated for biological pathway analysis. More than 5,600 significant differentially expressed genes (SDEGs) were identified across each comparison; the top 200 SDEGs exhibited greater than a two-fold expression change for each comparison except for RRMS/All Others. ML models utilizing supervised and unsupervised feature selection approaches produced consistent AUC values (0.75-0.82) and distinguished RRMS from NMO with up to 94% accuracy. Distinct gene expression profiles were also identified through cNMF. Sixteen genes distinguished RRMS and NMO across all methods and were associated with adaptive immune response, protein metabolism, cellular stress response, and nervous system development. Novel approaches for early diagnosis and treatment of demyelinating neurological disease represent an unmet clinical need. Through a multi-perspective, advanced analytics approach, our proof-of-concept studies identified candidate RNA biomarkers from whole blood to distinguish patients with RRMS or NMO. Future studies will validate these multivariate signatures in larger, prospective cohorts, including individuals with other inflammatory and non-inflammatory neurological diseases for novel diagnostic development.