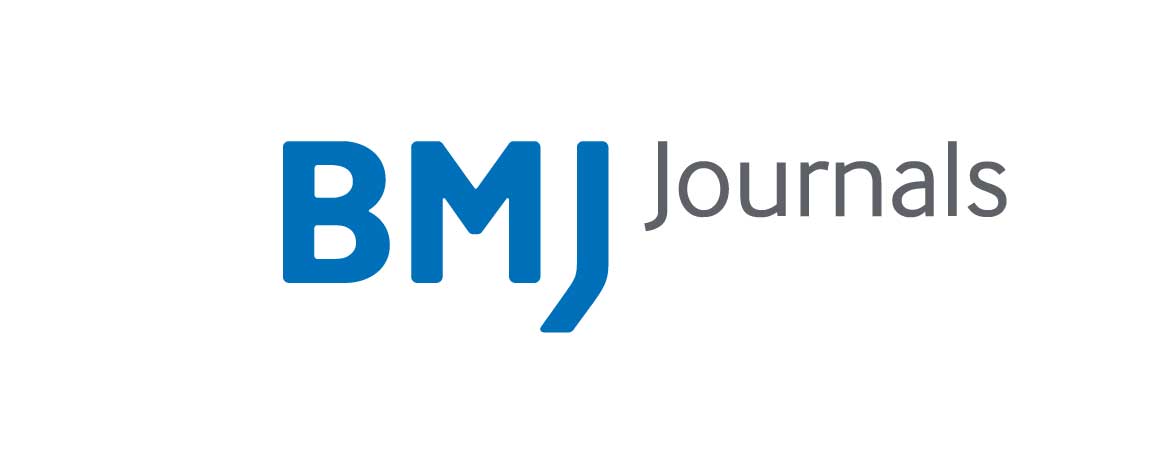
Predictive modeling of COVID-19 case growth highlights evolving racial and ethnic risk factors in Tennessee and Georgia
The SARS-CoV-2 (COVID-19) pandemic has exposed the need to understand the risk drivers that contribute to uneven morbidity and mortality in US communities. Addressing the community-specific social determinants of health (SDOH) that correlate with spread of SARS-CoV-2 provides an opportunity for targeted public health intervention to promote greater resilience to viral respiratory infections.
Abstract
Introduction The SARS-CoV-2 (COVID-19) pandemic has exposed the need to understand the risk drivers that contribute to uneven morbidity and mortality in US communities. Addressing the community-specific social determinants of health (SDOH) that correlate with spread of SARS-CoV-2 provides an opportunity for targeted public health intervention to promote greater resilience to viral respiratory infections.
Methods Our work combined publicly available COVID-19 statistics with county-level SDOH information. Machine learning models were trained to predict COVID-19 case growth and understand the social, physical and environmental risk factors associated with higher rates of SARS-CoV-2 infection in Tennessee and Georgia counties. Model accuracy was assessed comparing predicted case counts to actual positive case counts in each county.
Results The predictive models achieved a mean R2 of 0.998 in both states with accuracy above 90% for all time points examined. Using these models, we tracked the importance of SDOH data features over time to uncover the specific racial demographic characteristics strongly associated with COVID-19 incidence in Tennessee and Georgia counties. Our results point to dynamic racial trends in both states over time and varying, localized patterns of risk among counties within the same state. For example, we find that African American and Asian racial demographics present comparable, and contrasting, patterns of risk depending on locality.
Conclusion The dichotomy of demographic trends presented here emphasizes the importance of understanding the unique factors that influence COVID-19 incidence. Identifying these specific risk factors tied to COVID-19 case growth can help stakeholders target regional interventions to mitigate the burden of future outbreaks.
http://creativecommons.org/licenses/by-nc/4.0/
This is an open access article distributed in accordance with the Creative Commons Attribution Non Commercial (CC BY-NC 4.0) license, which permits others to distribute, remix, adapt, build upon this work non-commercially, and license their derivative works on different terms, provided the original work is properly cited, appropriate credit is given, any changes made indicated, and the use is non-commercial. See: http://creativecommons.org/licenses/by-nc/4.0/.