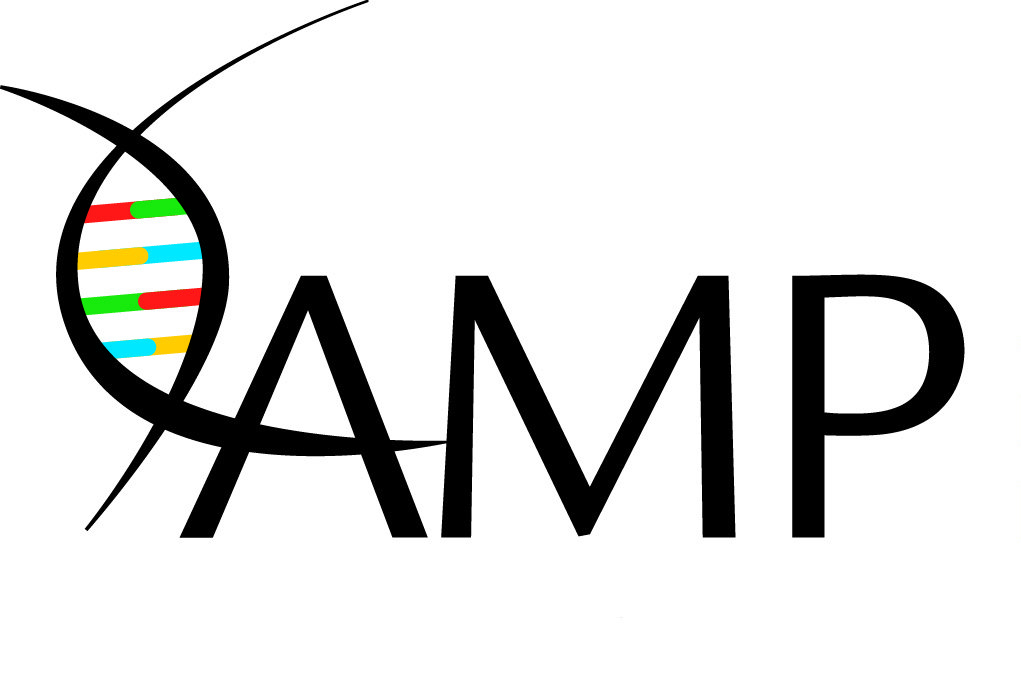
Application of an advanced data analytics platform leveraging artificial intelligence to identify RNA biomarkers
November 2023 – Poster Presentation
Lukasz S. Wylezinski1,2, Cheryl L. Sesler1, Jamieson D. Gray1, Guzel I. Shaginurova1, Elena V. Grigorenko1, Franklin R. Cockerill, III1,3, Michael J. McPhaul4, Michael K. Racke4, Charles F. Spurlock, III1,2,5
1 Decode Health, Nashville, TN, USA
2 Department of Medicine, Vanderbilt University School of Medicine, Nashville, TN 37203
3 Department of Medicine, Rush University Medical Center, Chicago, IL 60612
4 Quest Diagnostics, Secaucus, NJ, USA
5 Wagner School of Public Health, New York University, New York, NY 10012
Introduction. Exploration of next generation RNA sequencing data with advanced analytics leveraging artificial intelligence (AI) and machine learning (ML) is transforming the understanding of complex disease mechanisms. Though challenges remain in adopting these tools, AI-powered methods can interrogate large disparate datasets and lead to improved biomarker discovery for diagnostic and therapeutic applications. Here, we describe a scalable advanced analytics platform built with a robust quality control framework adaptable across multiple clinical indications and biospecimens (whole blood and cell-free plasma). In addition, we show our platform’s ability to identify disease-specific putative biomarkers ranging from proof-of-concept studies to larger studies employing ML analytics.
Methods. Total RNA sequencing was performed using RNA isolated from whole blood (PAXgene Blood RNA tubes) or plasma (Streck RNA Complete BCT). Sequences were aligned to the human genome and quantified. Advanced data analytics were leveraged to identify candidate biomarkers that were further interrogated for biological pathway associations. Differential gene expression was applied in an initial metabolic study assessing whole blood and plasma samples collected from insulin sensitive (IS, n=14) and insulin resistant (IR, n=14) cohorts. Application of the platform in a larger study focused on the identification of biomarkers to differentiate patients with diagnosed relapsing-remitting multiple sclerosis (RRMS, n=105), those with neuromyelitis optica (NMO, n=53), and healthy control patients (HC, n=69).
Results. We have leveraged our advanced data analytics platform in multiple clinical applications. Applying this platform in a metabolic study, we identified genes differentially expressed in both whole blood and plasma across IS and IR cohorts; these genes were associated with N-glycan synthesis, cellular senescence, and coagulation factor activity. In a larger independent study applying ML analytics, the best model was able to distinguish RRMS from NMO with 94% accuracy. Genes identified by these models were associated with ribosomal dysfunction, viral infection, and novel biological processes not typically associated with RRMS or NMO.
Conclusions. An advanced data analytics platform has been developed to accelerate biomarker discovery using RNA sequencing. This framework can accommodate different specimen and data types and could be implemented in clinical applications spanning diagnostics and therapeutic target discovery. The flexible platform permits integration of disparate biological, clinical, and environmental datasets agnostic to disease, cohort size, and enrichment dataset type, allowing unparalleled opportunities for biomarker discovery.